The 6th Korea-China-Japan Joint Visualization Workshop
Workshop Committee
Workshop co-Organizers:
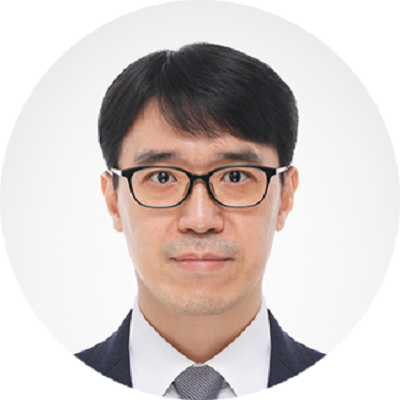
Yun Jang
Sejong University
South Korea
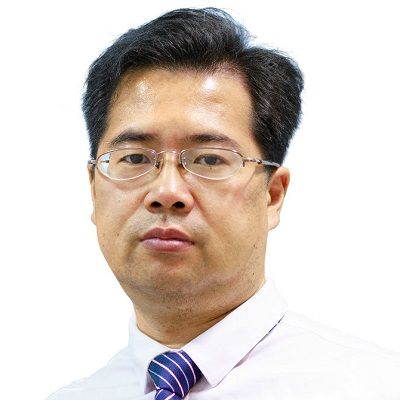
Xiaoru Yuan
Peking University
China
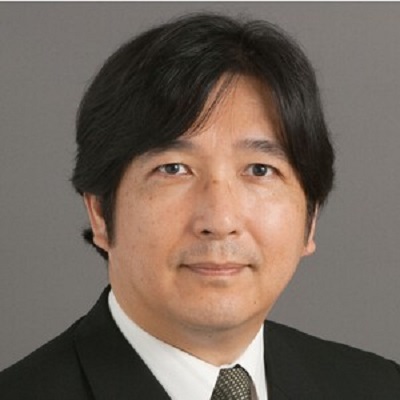
Issei Fujishiro
Keio University
Japan
In-Cooperation:
China Society of Image and Graphics - Visualization and Visual Analytics Technical Committee
Program (April 18, 2023)
Time | Speaker | |
---|---|---|
14:00 - 14:05 | Opening | |
14:05 - 14:30 | Takayuki Itoh | Recent Topics on Music Visualization |
14:30 - 14:55 | Shenghui Cheng | Graphical Enhancements for Effective Exemplar Identification in Multi-attribute Configuration Space |
14:55 - 15:20 | Isaac Cho | Distributed Data Visual Analytics Framework |
15:20 - 15:35 | Yu Liu | Revolutionizing Visual Analytics: Enhancing Human-AI Collaboration with Large Language Models |
15:35 - 16:00 | Break | |
16:00 - 16:25 | Yuri Miyagi | Visualization of Tuning History to Support Analysis of Machine Learning Models |
16:25 - 16:50 | Chansoo Kim | Non-Gaussian behaviors in a view of (information) flows and causality in a network |
16:50 - 17:30 | Shenghui Cheng, Yuri Miyagi, Takayuki Itoh, Issac Cho, Chansoo Kim, and Yu Liu | Panel |
17:30 - 17:35 | Closing |
14:00 - 14:05: Opening: Yun Jang (Sejong University, South Korea)
14:00 - 14:05: Recent Topics on Music Visualization
Speaker: Takayuki Itoh (Ochanomizu University, Japan)
Introduced by: Issei Fujishiro (Keio University, Japan)
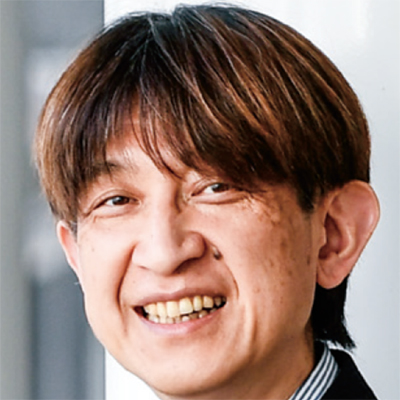
Bio:
Takayuki Itoh is a full professor at Ochanomizu University, Japan. He received his Ph.D. degree from
Waseda University in 1997. He was a researcher of IBM Research, Tokyo Research Laboratory from 1992
to 2005. He moved to Ochanomizu University as an associate professor in 2005 and then has been a full
professor since 2011. He is mainly working on information visualization, especially graph, hierarchical,
and multidimensional data visualization. He is the general chair of IEEE Pacific Visualization 2018,
Graph Drawing 2022, and short paper co-chair of IEEE VIS 2023.
Title:
Recent Topics on Music Visualization
Abstract:
Music is a typical content that is worth to be visualized. There have been two types of topics on music
visualization: visualization of the features or the structure of a single work, and visualization of
the distributions of a music collection. We can immediately understand the overall structure and
distribution of music by using visualizations while we need to take a time to listen to them. This talk
introduces typical studies on music visualization and then the speaker’s own recent studies on music
visualization.
14:30 - 14:55
Speaker: Shenghui Cheng (Westlake University, China)
Introduced by: Xiaoru Yuan (Peking University, China)
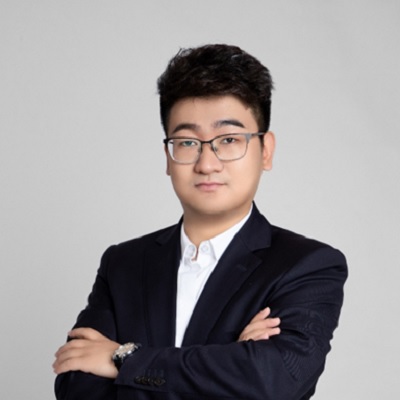
Bio:
Dr. Shenghui Cheng is a westlake fellow and the director of the Intelligent Visualization Lab, WestLake University, China. He obtained a Ph.D. in
computer science from Stony Brook University, and conducted research at Brookhaven National Laboratory and Harvard Medical School. He also
served as a consultant for the World Bank, US. His research areas are visualization and visual analytics with a special focus on high
dimensional data.
Title:
Graphical Enhancements for Effective Exemplar Identification in Multi-attribute Configuration Space
Abstract:
An exemplar is an entity that represents a desirable instance in a multi-attribute configuration space. It offers certain strengths in some of its
attributes without unduly compromising the strengths in other attributes. Exemplars are frequently sought after in real life applications,
such as systems engineering, investment banking, drug advisory, product marketing and many others. However, effective identifying the exemplar
in multi-attribute configuration spaces brings lots of challenge due to the high dimensionality and intricate relations inside the data.
In this talk, I will introduce a novel method for exemplar identification in multi-attribute configuration spaces based on the contextual
visualizations that produces a 2D embedding where users can view the data objects in the context of the data attributes. Then, certain graphical
enhancements are generated to help gain a better understanding of the attribute-wise tradeoffs and so select better exemplar sets. Three different
graphical designs, namely iso-contour, value-shaded topographic rendering and terrain topographic rendering, are proposed and compared with a
baseline DCM display with efficiently user studies.
14:55 - 15:20
Speaker: Isaac Cho (Utah State University, USA)
Introduced by: Yun Jang (Sejong University, South Korea)
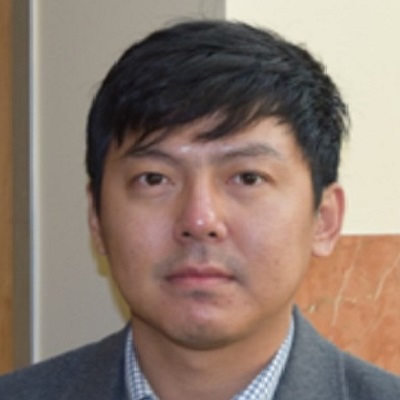
Bio:
Dr. Isaac Cho is an assistant professor in the Computer Science department at Utah State University.
He is also an adjunct professor in the Computer Science Department at the University of North Carolina
at Charlotte and an affiliated faculty member of the Ribarsky Center for Visual Analytics (the Charlotte
Visualization Center). His main research interests are Interactive Visual Analytics, Human-Computer
Interactions, 3D User Interfaces, and Virtual Environments. His research has been funded by NSF, DHS,
and EPRI. He has served as organizing and program committees of several international conferences such
as IEEE VR, IEEE Visualization, IEEE AIVR, and more. He received his Ph.D. in Computing and Information
Systems from UNC Charlotte in 2013.
Title:
Distributed Data Visual Analytics Framework
Abstract:
In this talk, I will present a visual analytics framework that addresses the complex user interactions
required through a command-line interface to run analyses in distributed data analysis systems.
The visual analytics framework facilitates the user to manage access to the distributed servers,
incorporate data from the source, run data-driven analysis, monitor the progress, and explore the
result using interactive visualizations. I will also show use cases utilizing the visual analytics
framework.
15:20 - 15:35
Speaker: Yu Liu (Xi'an Jiaotong-Liverpool University, China)
Introduced by: Xiaoru Yuan (Peking University, China)
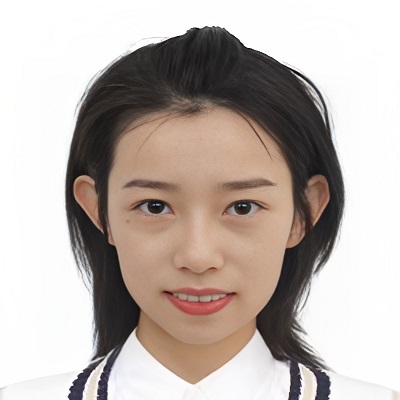
Bio:
Dr Yu Liu (刘钰) is a lecturer at the Department of Computing (CPT) at Xi’an Jiaotong-Liverpool University. She graduated from the
University of Liverpool in 2022. Dr Liu is a passionate researcher in the fields of Information Visualization (InfoViz) and Computer
Supported Cooperative Work (CSCW). Her research interests are in the design, evaluation, and application of InfoVis and CSCW in multi-device
settings, which involve a series of projects aimed at improving data visualization and collaboration practices.
Title:
Revolutionizing Visual Analytics: Enhancing Human-AI Collaboration with Large Language Models
Abstract:
Recent advances in Large Language Models (LLMs) have led to increased interest in leveraging these models to enhance human-AI collaboration in
visual analytics. LLMs provide a common language between humans and machines, facilitating communication and collaboration in visual analytics
workflows. They can also help bridge knowledge gaps between different stakeholders and enable more effective sharing of insights and recommendations.
In this talk, I will explore the benefits and challenges of integrating LLMs into visual analytics systems. I will discuss how LLMs can improve
natural language understanding, enabling more personalized insights and recommendations. I will also discuss some examples of implementations of
LLMs in visual analytics, including their use in generating natural language explanations and summaries of data, facilitating interactive and
conversational interfaces, and identifying hidden trends and anomalies in the data.
16:00 - 16:25: Visualization of Tuning History to Support Analysis of Machine Learning Models
Speaker: Yuri Miyagi (AIST, Japan)
Introduced by: Issei Fujishiro (Keio University, Japan)
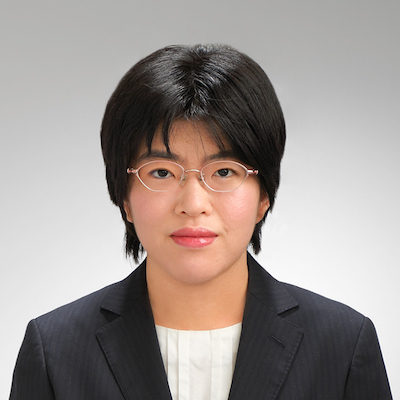
Bio:
Yuri Miyagi is a post-doctoral researcher at National Institute of Advanced Industrial Science and
Technology (AIST). She received her Ph.D. degree from Ochanomizu University in 2020. Her work focuses
on information visualization about human behaviors in society. Her recent work is developing a
visualization method to support the evaluation work of machine learning models.
Title:
Visualization of Tuning History to Support Analysis of Machine Learning Models
Abstract:
Machine learning technology continues to develop rapidly, and in some fields, high-performance models
have been created that make fewer errors than humans. On the other hand, it has also been shown that
human adjustments effectively improve the accuracy of machine learning models. It is significant for
model creators and users to be able to understand the internals and behavior of models, and visualization
for model characteristics or the flow of tunings is useful. This presentation introduces visualization
techniques that support the analysis of machine learning models and facilitate understanding of the models.
16:25 - 16:50
Speaker: Chansoo Kim (Korea Institute of Science and Technology (KIST), South KOrea)
Introduced by: Yun Jang (Sejong University, South Korea)
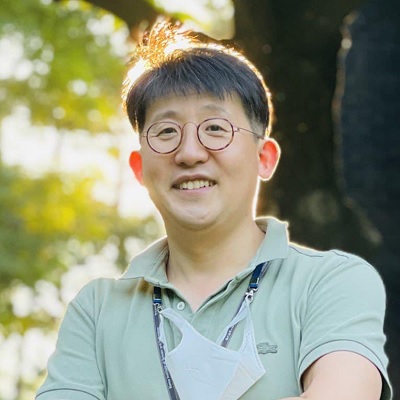
Bio:
Chansoo Kim is a research scientist as well as an economist at the Computational Science Research Center
in Korea Institute of Science and Technology (KIST) in Republic of Korea. He leads the group
‘Information Machine Learning Financial Econ Lab’, which works on the science of information and
complex (adaptive) systems.
His research focuses on heavy-tailed distributions such as leptokurtic distribution, which are among
the non-Gaussian behaviours, and its applications to learning, reasoning, finance as well as inequality.
One of his research and academic ancestors is C. F. Gauß. He has been working hard to be a good scientist,
who stands under the intellectual tradition of Neruda, Szymborska, Lacan, Levinas, Hirofumi, Keynes,
Mandelbrot, Boulding, Prigogine, Shannon and Boltzmann.
For the COVID-19 pandemic, his group has performed a series of macroscale individual-based simulations
for policy development and assessments with the Korean government using parallel computers. He obtained
his B.Sc. in Computer Science and M.A. focusing on Statistical Physics, and his PhD is in Economics
(Mathematical Finance and Machine Learning).
Title:
Non-Gaussian behaviors in a view of (information) flows and causality in a network
Abstract:
Solutions of the heat equation are obtained in a form of combined kernel polynomials. They possibly
provide a series of non-Gaussian behaviors, which observed in many cases. The equation also carries
many solutions under given boundary conditions. It shows the (information) flows, and they work as a
ground to how to interpret the causality in a network. The entropy borne from these solutions is also
computed and it shows the deviation from the Gaussian one.
16:50 - 17:30: Panel
ModeratorXiaoru Yuan, Issei Fujishiro
Panellist
- Shenghui Cheng
- Yuri Miyagi
- Takayuki Itoh
- Issac Cho
- Chansoo Kim
- Yu Liu
Topics
- VIS roles for (X)AI in the future
- Impact of the large language model (LLM) on VIS
- LLM and multi-modality for future VIS interaction research