VisMeetsAI Workshop Program
(April 18, 2023)
Session | Time | Speaker | |
---|---|---|---|
Session 1 (Chair: Junpeng Wang) |
9:30 - 10:30 | Andreas Kerren | Invited Talk 1 |
10:30 - 10:50 | Hsiao-Ying Lu et al. | Paper Presentation 1 | |
Break | 10:50 - 11:10 | ||
Session 2 (Chair: Takanori Fujiwara) |
11:10 - 12:10 | Sungahn Ko | Invited Talk 2 |
12:10 - 12:30 | Syu-Ting Deng et al. | Paper Presentation 2 |
9:30 - 10:50 Session 1 (Chair: Junpeng Wang)
9:30 - 10:30: Invited Talk 1:
Professor Andreas Kerren (Linköping University and Linnaeus University, Sweden)
9:30 - 10:30: Invited Talk 1: Professor Andreas Kerren (Linköping University and Linnaeus University, Sweden)
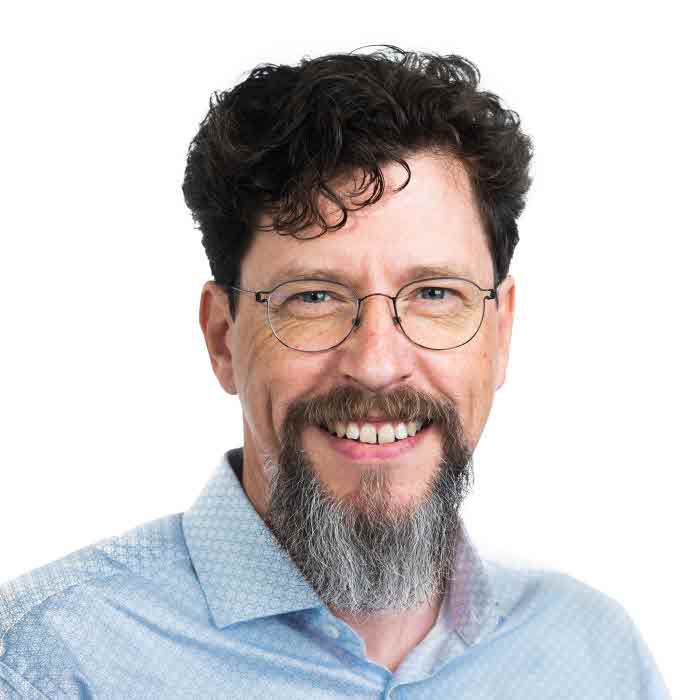
Bio:
Prof. Dr. Andreas Kerren received his PhD degree in Computer Science from Saarland University,
Saarbrücken, Germany. In 2008, he achieved his habilitation (docent competence) from Växjö University,
Sweden. Dr. Kerren is currently a Full Professor of Information Visualization, Linköping University
(LiU) and Linnaeus University (LNU), Sweden. He holds the Chair of Information Visualization at LiU
and is head of the research group Information and Software Visualization at LNU. In addition, he is an
ELLIIT professor supported by the Excellence Center at Linköping–Lund in Information Technology and key
researcher of the Linnaeus University Centre for Data Intensive Sciences and Applications. His main
research interests include several areas of information visualization and visual analytics, especially
visual network analytics, text visualization, and the use of visual analytics for explainable AI.
He has been editorial board member of a number of journals such as Information Visualization or Computer
Graphics Forum, has served as organizer/program chair at numerous conferences such as IEEE VISSOFT
2013/2018 or GD 2018, and has edited a number of successful books on human-centered visualization.
Dr. Kerren has published more than 200 peer-reviewed papers, articles, and book chapters.
Title:
Visualization Perspectives in Explainable and Trustworthy Machine Learning
Abstract:
This talk will overview interactive data visualization research with a focus on the development and
use of visualization techniques for explainable and trustworthy machine learning. As supervised and
unsupervised machine learning are both considered as complex and their internal operations are mostly
hidden in black boxes, it becomes difficult for model developers but also for analysts to assess and
trust their results. Moreover, choosing appropriate machine learning algorithms or setting hyperparameters
are further challenges where the human in the loop is necessary. I will exemplify solutions of some of
these challenges with the help of a selection of visualization showcases recently developed by the iVis
research group at Linköping University and the ISOVIS research group at Linnaeus University, Sweden.
Our visual analytics examples range from the visual exploration of the most performant and most diverse
models for the creation of stacking ensembles, the use of multiple embeddings for data analysis and
visualization, to ideas of making the black boxes of complex dimensionality reduction techniques more
transparent in order to increase the trust into their results. Moreover, I will highlight the most
recent analytical results of our state-of-the-art reports on visualization for enhancing trust into
machine learning and the use of embeddings in visual analytics. My talk will conclude with a discussion
of the most important trends and challenges in VIS4AI and AI4VIS. All together I hope that this talk
can serve as a starting point for further discussions during the PacificVis 2023 Visualization Meets
AI Workshop.
10:30 - 10:50 Paper Presentations 1
11:10 - 12:30 Session 2 (Chair: Takanori Fujiwara)
11:10 - 12:10: Invited Talk 2: Professor Sungahn Ko (UNIST, South Korea)
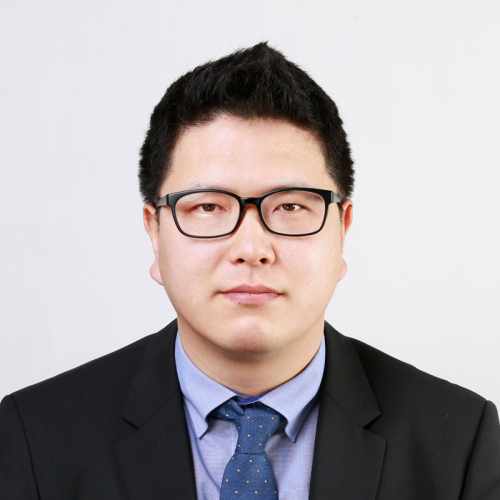
Bio:
Sungahn Ko is an associate professor at the School of Computer Science and Engineering and Graduate
School of Artificial Intelligence at UNIST in Ulsan, South Korea. His research interests include
visual analytics, information visualization, deep learning, and human-computer interaction (HCI) with
a focus on human-AI collaboration. His work has been published in premier venues in visualization,
HCI, and AI, including IEEE VIS, IEEE TVCG, and ACM SIGCHI, CIKM, and ICLR. He has also served as a
program committee member for IEEE VIS and PacificVis. He received a doctoral degree in electrical and
computer engineering from Purdue University in 2014, an M.S. from KAIST in 2008, and a B.E. from Korea
University in 2006. For more information, please visit https://ivader.unist.ac.kr/home.
Title:
Visualization Meets AI for Future Mobility
Abstract:
As mobility systems comprise important societal infrastructure that is directly relevant to our lives,
system inefficiency can cause stressful situations and waste societal resources. For example, sudden
traffic congestion affects drivers not only on the congested road but also on adjacent roads due to
congestion propagation. Likewise, ineffective geographical distribution of taxis in a city can increase
passenger wait times and waste resources. In this talk, I present how visualization can support mobility
system experts. First, I introduce a visual analytics system that allows a city’s traffic control experts
to analyze, monitor, and predict future traffic speeds at work. To accurately predict the future traffic
conditions of a city’s road network, it is important to understand the behaviors of AI models. From this
perspective, I describe how a visualization system can help people explore the behaviors of a deep
learning model and utilize the analysis results for proving improved navigation services. Lastly,
I discuss important tasks in the mobility domain, such as mobility-on-demand, that are underexplored
via visualization.